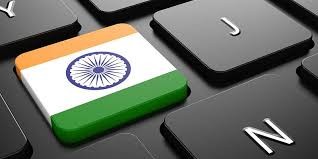
Personal Data Sharing & Protection: Strategic relevance from India’s context
Add Your Heading Text Here
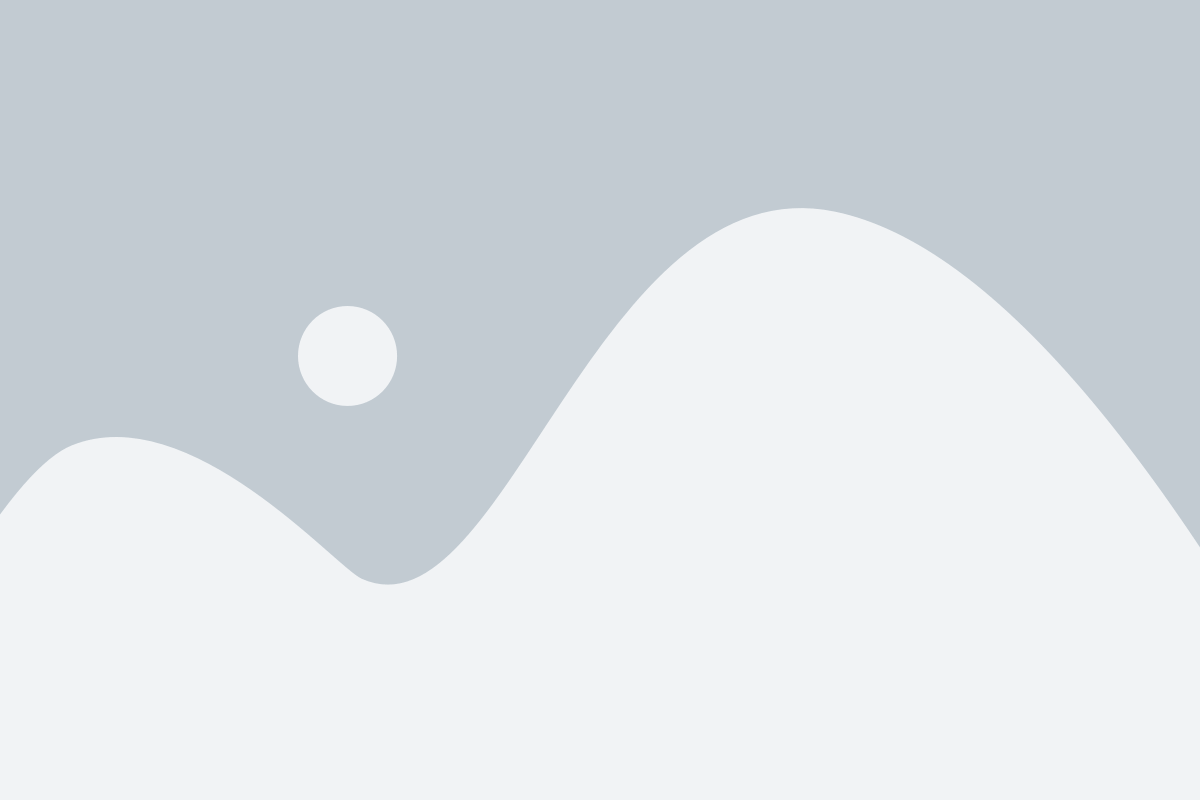
India’s Investments in the digital financial infrastructure—known as “India Stack”—have sped up the large-scale digitization of people’s financial lives. As more and more people begin to conduct transactions online, questions have emerged about how to provide millions of customers adequate data protection and privacy while allowing their data to flow throughout the financial system. Data-sharing among financial services providers (FSPs) can enable providers to more efficiently offer a wider range of financial products better tailored to the needs of customers, including low-income customers.
However, it is important to ensure customers understand and consent to how their data are being used. India’s solution to this challenge is account aggregators (AAs). The Reserve Bank of India (RBI) created AAs in 2018 to simplify the consent process for customers. In most open banking regimes, financial information providers (FIPs) and financial information users (FIUs) directly exchange data. This direct model of data exchange—such as between a bank and a credit bureau—offers customers limited control and visibility into what data are being shared and to what end. AAs have been designed to sit between FIPs and FIUs to facilitate data exchange more transparently. Despite their name, AAs are barred from seeing, storing, analyzing, or using customer data. As trusted, impartial intermediaries, they simply manage consent and serve as the pipes through which data flow among FSPs. When a customer gives consent to a provider via the AA, the AA fetches the relevant information from the customer’s financial accounts and sends it via secure channels to the requesting institution. implementation of its policies for consensual data-sharing, including the establishment and operation of AAs. It provides a set of guiding design principles, outlines the technical format of data requests, and specifies the parameters governing the terms of use of requested data. It also specifies how to log consent and data flows.
There are several operational and coordination challenges across these three types of entities: FIPs, FIUs, and AAs. There are also questions around the data-sharing business model of AAs. Since AAs are additional players, they generate costs that must be offset by efficiency gains in the system to mitigate overall cost increases to customers. It remains an open question whether AAs will advance financial inclusion, how they will navigate issues around digital literacy and smartphone access, how the limits of a consent-based model of data protection and privacy play out, what capacity issues will be encountered among regulators and providers, and whether a competitive market of AAs will emerge given that regulations and interoperability arrangements largely define the business.
Account Aggregators (AA’s):
ACCOUNT AGGREGATORS (AAs) is one of the new categories of non banking financial companies (NBFCs) to figure into India Stack—India’s interconnected set of public and nonprofit infrastructure that supports financial services. India Stack has scaled considerably since its creation in 2009, marked by rapid digitization and parallel growth in mobile networks, reliable data connectivity, falling data costs, and continuously increasing smartphone use. Consequently, the creation, storage, use, and analyses of personal data have become increasingly relevant. Following an “open banking “approach, the Reserve Bank of India (RBI) licensed seven AAs in 2018 to address emerging questions around how data can be most effectively leveraged to benefit individuals while ensuring appropriate data protection and privacy, with consent being a key element in this. RBI created AAs to address the challenges posed by the proliferation of data by enabling data-sharing among financial institutions with customer consent. The intent is to provide a method through which customers can consent (or not) to a financial services provider accessing their personal data held by other entities. Providers are interested in these data, in part, because information shared by customers, such as bank statements, will allow providers to better understand customer risk profiles. The hypothesis is that consent-based data-sharing will help poorer customers qualify for a wider range of financial products—and receive financial products better tailored to their needs.
Data Sharing Model : The new perspective:
Paper based data collection is inconvenient , time consuming and costly for customers and providers. Where models for digital-sharing exist, they typically involve transferring data through intermediaries that are not always secure or through specialized agencies that offer little protection for customers. India’s consent-based data-sharing model provides a digital framework that enables individuals to give and withdraw consent on how and how much of their personal data are shared via secure and standardized channels. India’s guiding principles for sharing data with user consent—not only in the financial sector— are outlined in the National Data Sharing and Accessibility Policy (2012) and the Policy for Open Application Programming Interfaces for the Government of India. The Information Technology Act (2000) requires any entity that shares sensitive personal data to obtain consent from the user before the information is shared. The forthcoming Personal Data Protection Bill makes it illegal for institutions to share personal data without consent.
India’s Ministry of Electronics and Information Technology (MeitY) has issued an Electronic Consent Framework to define a comprehensive mechanism to implement policies for consensual data-sharing. It provides a set of guiding design principles, outlines the technical format of the data request, and specifies the parameters governing the terms of use of the data requested. It also specifies how to log both consent and data flows. This “consent artifact” was adopted by RBI, SEBI, IRDAI, and PFRDA. Components of the consent artifact structure include :
- Identifier : Specifies entities involved in the transaction: who is requesting the data, who is granting permission, who is providing the data, and who is recording consent.
- Data : Describes the type of data being accessed and the permissions for use of the data. Three types of permissions are available: view (read only), store, and query (request for specific data). The artifact structure also specifies the data that are being shared, date range for which they are being requested, duration of storage by the consumer, and frequency of access.
- Purpose : Describes end use, for example, to compute a loan offer.
- Log : Contains logs of who asked for consent, whether it was granted or not, and data flows.
- Digital signature : Identifies the digital signature and digital ID user certificate used by the provider to verify the digital signature. This allows providers to share information in encrypted form
The Approach :
THE AA consent based data sharing model mediates the flow of data between producers and users of data, ensuring that sharing data is subject to granular customer consent. AAs manage only the consent and data flow for the benefit of the consumer, mitigating the risk of an FIU pressuring consumers to consent to access to their data in exchange for a product or service. However, AAs, as entities that sit in the middle of this ecosystem, come with additional costs that will affect the viability of the business model and the cost of servicing consumers. FIUs most likely will urge consumers to go directly to an AA to receive fast, efficient, and low-cost services. However, AAs ultimately must market their services directly to the consumer. While AA services are not an easy sell, the rising levels of awareness among Indian consumers that their data are being sold without their consent or knowledge may give rise to the initial wave of adopters. While the AA model is promising, it remains to be seen how and when it will have a direct impact on the financial lives of consumers.
Differences between Personal Data Protection & GDPR ?
There are some major differences between the two.
First, the bill gives India’s central government the power to exempt any government agency from the bill’s requirements. This exemption can be given on grounds related to national security, national sovereignty, and public order.
While the GDPR offers EU member states similar escape clauses, they are tightly regulated by other EU directives. Without these safeguards, India’s bill potentially gives India’s central government the power to access individual data over and above existing Indian laws such as the Information Technology Act of 2000, which dealt with cyber crime and e-commerce.
Second, unlike the GDPR, India’s bill allows the government to order firms to share any of the non personal data they collect with the government. The bill says this is to improve the delivery of government services. But it does not explain how this data will be used, whether it will be shared with other private businesses, or whether any compensation will be paid for the use of this data.
Third, the GDPR does not require businesses to keep EU data within the EU. They can transfer it overseas, so long as they meet conditions such as standard contractual clauses on data protection, codes of conduct, or certification systems that are approved before the transfer.
The Indian bill allows the transfer of some personal data, but sensitive personal data can only be transferred outside India if it meets requirements that are similar to those of the GDPR. What’s more, this data can only be sent outside India to be processed; it cannot be stored outside India. This will create technical issues in delineating between categories of data that have to meet this requirement, and add to businesses’ compliance costs.
Related Posts
AIQRATIONS
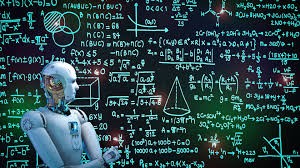
AI Strategy: The Epiphany of Digital Transformation
Add Your Heading Text Here
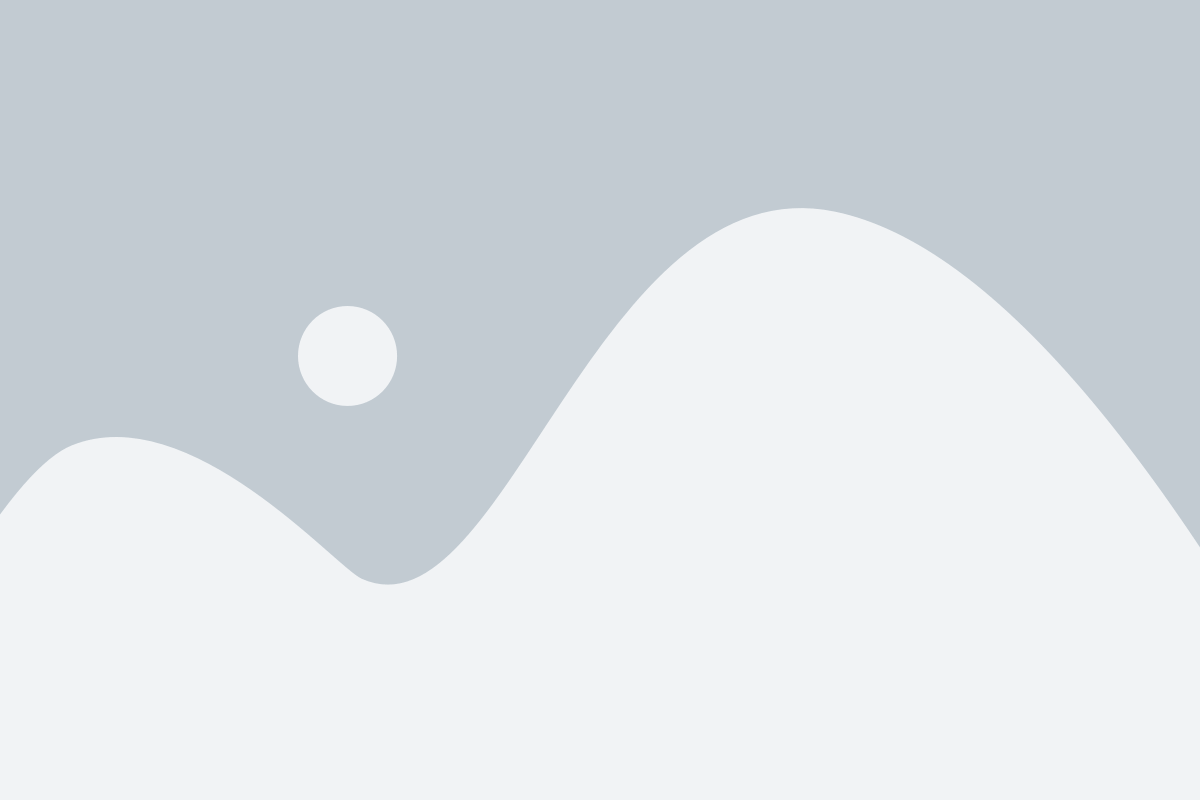
In the past months due to lockdowns and WFH, enterprises have got an epiphany of massive shifts of business and strategic models for staying relevant and solvent. Digital transformation touted as the biggest strategic differentiation and competitive advantages for enterprises faced a quintessential inertia of mass adoption in the legacy based enterprises and remained more on business planning slides than in full implementation. However, Digital Transformation is not about aggregation of exponential technologies and adhoc use cases or stitching alliances with deep tech startups. The underpinning of Digital transformation is AI and how AI strategy has become the foundational aspect of accomplishing digital transformation for enterprises and generating tangible business metrics. But before we get to the significance of AI strategy in digital transformation, we need to understand the core of digital transformation itself. Because digital transformation will look different for every enterprise, it can be hard to pinpoint a definition that applies to all. However, in general terms: we define digital transformation as the integration of core areas of business resulting in fundamental changes to how businesses operate and how they deliver value to customers.
Though, in specific terms digital transformation can take a very interesting shape according to the business moment in question. From a customer’s point of view, “Digital transformation closes the gap between what digital customers already expect and what analog businesses actually deliver.”
Does Digital Transformation really mean bunching exponential technologies? I believe that digital transformation is first and foremost a business transformation. Digital mindset is not only about new age technology, but about curiosity, creativity, problem-solving, empathy, flexibility, decision-making and judgment, among others. Enterprises needs to foster this digital mindset, both within its own boundaries and across the company units. The World Economic Forum lists the top 10 skills needed for the fourth industrial revolution. None of them is totally technical. They are, rather, a combination of important soft skills relevant for the digital revolution. You don’t need to be a technical expert to understand how technology will impact your work. You need to know the foundational aspects, remain open-minded and work with technology mavens. Digital Transformation is more about cultural change that requires enterprises to continually challenge the status quo, experiment often, and get comfortable with failure. The most likely reason for business to undergo digital transformation is the survival & relevance issue. Businesses mostly don’t transform by choice because it is expensive and risky. Businesses go through transformation when they have failed to evolve. Hence its implementation calls for tough decisions like walking away from long-standing business processes that companies were built upon in favor of relatively new practices that are still being defined.
Business Implementation aspects of Digital Transformation
Disruption in digital business implies a more positive and evolving atmosphere, instead of the usual negative undertones that are attached to the word. According to the MIT Center for Digital Business, “Companies that have embraced digital transformation are 26 percent more profitable than their average industry competitors and enjoy a 12 percent higher market valuation.” A lot of startups and enterprises are adopting an evolutionary approach in transforming their business models itself, as part of the digital transformation. According to Mckinsey, One-third of the top 20 firms in industry segments will be disrupted by new competitors within five years.
The various Business Models being adopted in Digital Transformation era are:
- The Subscription Model (Netflix, Dollar Shave Club, Apple Music) Disrupts through “lock-in” by taking a product or service that is traditionally purchased on an ad hoc basis, and locking-in repeat custom by charging a subscription fee for continued access to the product/service
- The Freemium Model (Spotify, LinkedIn, Dropbox) Disrupts through digital sampling, where users pay for a basic service or product with their data or ‘eyeballs’, rather than money, and then charging to upgrade to the full offer. Works where marginal cost for extra units and distribution are lower than advertising revenue or the sale of personal data
- The Free Model (Google, Facebook) Disrupts with an ‘if-you’re-not-paying-for-the-product-you-are-the-product’ model that involves selling personal data or ‘advertising eyeballs’ harvested by offering consumers a ‘free’ product or service that captures their data/attention
- The Marketplace Model (eBay, iTunes, App Store, Uber, Airbnb) Disrupts with the provision of a digital marketplace that brings together buyers and sellers directly, in return for a transaction or placement fee or commission
- The Access-over-Ownership Model (Zipcar, Peer buy) Disrupts by providing temporary access to goods and services traditionally only available through purchase. Includes ‘Sharing Economy’ disruptors, which takes a commission from people monetizing their assets (home, car, capital) by lending them to ‘borrowers’
- The Hypermarket Model (Amazon, Apple) Disrupts by ‘brand bombing’ using sheer market power and scale to crush competition, often by selling below cost price
- The Experience Model (Tesla, Apple) Disrupts by providing a superior experience, for which people are prepared to pay
- The Pyramid Model (Amazon, Microsoft, Dropbox) Disrupts by recruiting an army of resellers and affiliates who are often paid on a commission-only mode
- The On-Demand Model (Uber, Operator, TaskRabbit) Disrupts by monetizing time and selling instant-access at a premium. Includes taking a commission from people with money but no time who pay for goods and services delivered or fulfilled by people with time but no money
- The Ecosystem Model (Apple, Google) Disrupts by selling an interlocking and interdependent suite of products and services that increase in value as more are purchased. Creates consumer dependency
Since Digital Transformation and its manifestation into various business models are being fast adopted by startups, there are providing tough competition to incumbent corporate houses and large enterprises. Though enterprises are also looking forward to digitally transform their enterprise business, the scale and complexity makes it difficult and resource consuming activity. It has imperatively invoked the enterprises to bring certain strategy to counter the cannibalizing effect in the following ways:
- The Block Strategy. Using all means available to inhibit the disruptor. These means can include claiming patent or copyright infringement, erecting regulatory hurdles, and using other legal barriers.
- The Milk Strategy. Extracting the most value possible from vulnerable businesses while preparing for the inevitable disruption
- The Invest in Disruption Model. Actively investing in the disruptive threat, including disruptive technologies, human capabilities, digitized processes, or perhaps acquiring companies with these attributes
- The Disrupt the Current Business Strategy. Launching a new product or service that competes directly with the disruptor, and leveraging inherent strengths such as size, market knowledge, brand, access to capital, and relationships to build the new business
- The Retreat into a Strategic Niche Strategy. Focusing on a profitable niche segment of the core market where disruption is less likely to occur (e.g. travel agents focusing on corporate travel, and complex itineraries, book sellers and publishers focusing on academia niche)
- The Redefine the Core Strategy. Building an entirely new business model, often in an adjacent industry where it is possible to leverage existing knowledge and capabilities (e.g. IBM to consulting, Fujifilm to cosmetics)
- The Exit Strategy. Exiting the business entirely and returning capital to investors, ideally through a sale of the business while value still exists (e.g. MySpace selling itself to Newscorp)
The curious evolution of AI and its relevance in digital transformation
So here’s an interesting question, AI has been around for more than 60 years, then why is it that it is only gaining traction with the advent of digital? The first practical application of such “machine intelligence” was introduced by Alan Turing, British mathematician and WWII code-breaker, in 1950. He even created the Turing test, which is still used today, as a benchmark to determine a machine’s ability to “think” like a human.The biggest differences between AI then and now are Hardware limitations, access to data, and rise of machine learning.
Hardware limitations led to the non-sustenance of AI adoption till late 1990s. There were many instances where the scope and opportunity of AI led transformation was identified and appreciated by implementation saw more difficult circumstances. The field of AI research was founded at a workshop held on the campus of Dartmouth College during the summer of 1956. But Eventually it became obvious that they had grossly underestimated the difficulty of the project due to computer hardware limitations. The U.S. and British Governments stopped funding undirected research into artificial intelligence, leading to years known as an “AI winter”.
In another example, again in 1980, a visionary initiative by the Japanese Government inspired governments and industry to provide AI with billions of dollars, but by the late 80s the investors became disillusioned by the absence of the needed computer power (hardware) and withdrew funding again. Investment and interest in AI boomed in the first decades of the 21st century, when machine learning was successfully applied to many problems in academia and industry due to the presence of powerful computer hardware. Teaming this with the rise in digital, leading to an explosion of data and adoption of data generation in every aspect of business, made it highly convenient for AI to not only be adopted but to evolve to more accurate execution.
The Core of Digital Transformation: AI Strategy
According to McKinsey, by 2023, 85 percent of all digital transformation initiatives will be embedded with AI strategy at its core. Due to radical computational power, near-endless amounts of data, and unprecedented advances in ML algorithms, AI strategy will emerge as the most disruptive business scenario, and its manifestation into various trends that we see and will continue to see, shall drive the digital transformation as we understand it. The following will the future forward scenarios of AI strategy becoming core to digital transformation:
AI’s growing entrenchment: This time, the scale and scope of the surge in attention to AI is much larger than before. For starters, the infrastructure speed, availability, and sheer scale has enabled bolder algorithms to tackle more ambitious problems. Not only is the hardware faster, sometimes augmented by specialized arrays of processors (e.g., GPUs), it is also available in the shape of cloud services , data farms and centers
Geography, societal Impact: AI adoption is reaching institutions outside of the industry. Lawyers will start to grapple with how laws should deal with autonomous vehicles; economists will study AI-driven technological unemployment; sociologists will study the impact of AI-human relationships. This is the world of the future and the new next.
Artificial intelligence will be democratized: As per the results of a recent Forrester study , it was revealed that 58 percent of professionals researching artificial intelligence ,only 12 percent are actually using an AI system. Since AI requires specialized skills or infrastructure to implement, Companies like Facebook have realized this and are already doing all they can to simplify the implementation of AI and make it more accessible. Cloud platforms like Google APIs, Microsoft Azure, AWS are allowing developers to create intelligent apps without having to set up or maintain any other infrastructure.
Niche AI will Grow: By all accounts, 2020 & beyond won’t be for large, general-purpose AI systems. Instead, there will be an explosion of specific, highly niche artificial intelligence adoption cases. These include autonomous vehicles (cars and drones), robotics, bots (consumer-orientated such as Amazon Echo , and industry specific AI (think finance, health, security etc.).
Continued Discourse on AI ethics, security & privacy: Most AI systems are immensely complex sponges that absorb data and process it at tremendous rates. The risks related to AI ethics, security and privacy are real and need to be addressed through consideration and consensus. Sure, it’s unlikely that these problems will be solved in 2020, but as long as the conversation around these topics continues, we can expect at least some headway.
Algorithm Economy: With massive data generation using flywheels, there will be an economy created for algorithms, like a marketplace for algorithms. The engineers, data scientists, organizations, etc. will be sharing algorithms for using the data to extract required information set.
Where is AI Heading in the Digital Road?While much of this is still rudimentary at the moment, we can expect sophisticated AI to significantly impact our everyday lives. Here are four ways AI might affect us in the future:
Humanizing AI: AI will grow beyond a “tool” to fill the role of “co-worker.” Most AI software is too hidden technologically to significantly change the daily experience for the average worker. They exist only in a back end with little interface with humans. But several AI companies combine advanced AI with automation and intelligent interfaces that drastically alter the day to day workflow for workers
Design Thinking & behavioral science in AI: We will witness Divergence from More Powerful Intelligence To More Creative Intelligence. There have already been attempts to make AI engage in creative efforts, such as artwork and music composition. we’ll see more and more artificial intelligence designing artificial intelligence, resulting in many mistakes, plenty of dead ends, and some astonishing successes.
Rise of Cyborgs: As augmented AI is already the mainstream thinking; the future might hold witness to perfect culmination of man-machine augmentation. AI augmented to humans, intelligently handling operations which human cannot do, using neural commands.
AI Oracle : AI might become so connected with every aspect of our lives, processing though every quanta of data from every perspective that it would perfectly know how to raise the overall standard of living for the human race. People would religiously follow its instructions (like we already follow GPS navigations) leading to leading to an equation of dependence closer to devotion.
The Final Word
Digital business transformation is the ultimate challenge in change management. It impacts not only industry structures and strategic positioning, but it affects all levels of an organization (every task, activity, process) and even its extended supply chain. Hence to brace Digital led disruption, one has to embrace AI-led strategy. Organizations that deploy AI strategically will ultimately enjoy advantages ranging from cost reductions and higher productivity to top-line benefits such as increasing revenue and profits, richer customer experiences, and working-capital optimization.
( AIQRATE, A bespoke global AI advisory and consulting firm. A first in its genre, AIQRATE provides strategic AI advisory services and consulting offerings across multiple business segments to enable clients navigate their AI powered transformation, innovation & revival journey and accentuate their decision making and business performance.
AIQRATE works closely with Boards, CXOs and Senior leaders advising them on their Analytics to AI journey construct with the art of possible AI roadmap blended with a jump start approach to AI driven transformation with AI@scale centric strategy; AIQRATE also consults on embedding AI as core to business strategy within business processes & functions and augmenting the overall decision-making capabilities. Our bespoke AI advisory services focus on curating & designing building blocks of AI strategy, embed AI@scale interventions and create AI powered organizations.
AIQRATE’s path breaking 50+ AI consulting frameworks, methodologies, primers, toolkits and playbooks crafted by seasoned and proven AI strategy advisors enable Indian & global enterprises, GCCs, Startups, SMBs, VC/PE firms, and Academic Institutions enhance business performance & ROI and accelerate decision making capability. AIQRATE also provide advisory support to Technology companies, business consulting firms, GCCs, AI pure play outfits on curating discerning AI capabilities, solutions along with differentiated GTM and market development strategies.
Visit www.aiqrate.ai to experience our AI advisory services & consulting offerings. Follow us on Linkedin | Facebook | YouTube | Twitter | Instagram )
Related Posts
AIQRATIONS
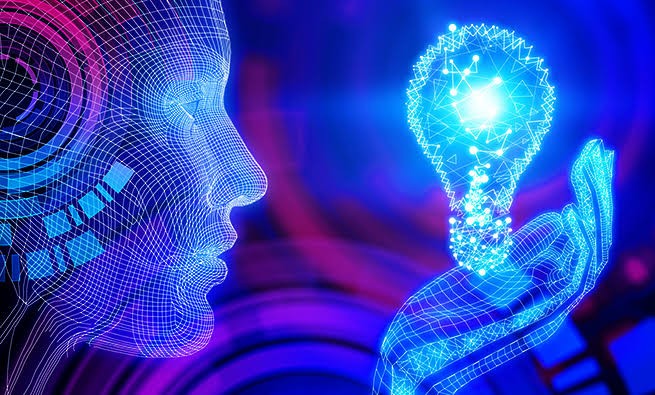
AI for Strategic Innovation
Add Your Heading Text Here
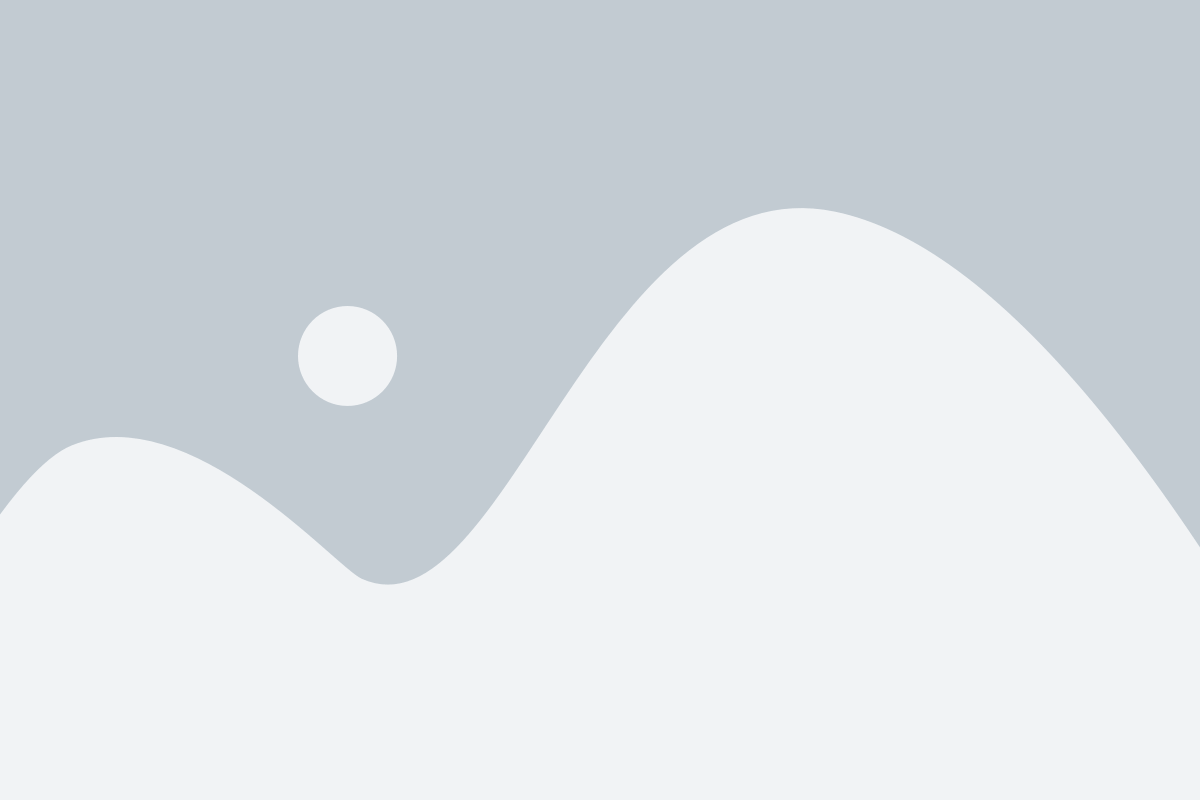
The extra ordinary promise of AI : Global & Indian enterprises have a lot to gain from unleashing innovation with AI —but harnessing their potential demands focused investment and a new way of working with external partners.
Here are few salient features of how AI has become game changing trend in spurring innovation; existing challenges and few strategic approaches of unlocking innovation with AI :
- 22% growth : From 2015 through 2019, disclosed private investment in seven deep tech sectors grew an average of 22% per year, equaling nearly $60 billion in total investment. Corporate venture capital is also playing an increasingly active role.
- Total investment : Nearly $60 Billion Invested in Deep Tech’s Fastest-Growing Sectors in 2019; Artificial intelligence corners close to $25 Bn
- About 1800 AI led startups in the US accounted for roughly half of this total investment, but other countries are catching up fast.
Existing Challenges
- Complex ecosystems : Multiple types of players including startups, venture capital firms, governments, universities and research centers, and early-adopter user groups
- Dynamic Interactions : Few central orchestrators; business relationships based on informal networks rather than formal contracts
Strategic approaches of unlocking innovation with AI :
- Cooperate in order to compete : Think beyond the enterprise’s immediate goals; commit to a long-term vision for the development of the ecosystem as whole
- Identify capabilities that add value : Define what the enterprise can offer to nurture the ecosystem and bring AI to market—not only money but also access to customers, data, networks, mentors, and technical experts
- Don’t pick winners in advance : AI startups are evolving rapidly. Continuously monitor the ecosystem to identify successful startups, applications, and business models as they emerge
- Blur the boundaries with partners : Make it easy for AI partners to navigate your corporate system. Define a clear role for them in your innovation strategy, ensure senior-executive sponsorship, and engage the core businesses
- Streamline decision making and governance : Success requires partnering more nimbly with fast-moving AI startups. Embrace agile ways of working.
- Develop breakthrough solutions by combining expertise from previously unconnected fields or industries. Be alert for game hanging opportunities that deliver both economic and social value.
AI will transform business and society in the future. The time to craft a AI strategy for unleashing innovation is now.
AIQRATE works closely with global & Indian enterprises , GCCs , VC/PE firms and has an extensive yet curated database of 1000 + global AI startups , boutique and niche firms benchmarked on our “Glow Curve” assessment.
(AIQRATE advisory & consulting is a bespoke AI advisory and consulting firm and provide strategic advisory services to boards , CXOs, senior leaders to curate , design building blocks of AI strategy , embed AI@scale interventions and create AI powered enterprises . visit : www.aiqrate.ai ; reach out to us at consult@aiqrate.ai )
Related Posts
AIQRATIONS
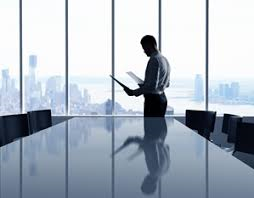
Introducing AIQRATE’s bespoke consulting offerings for CHRO/CPO/HR Leaders
Add Your Heading Text Here
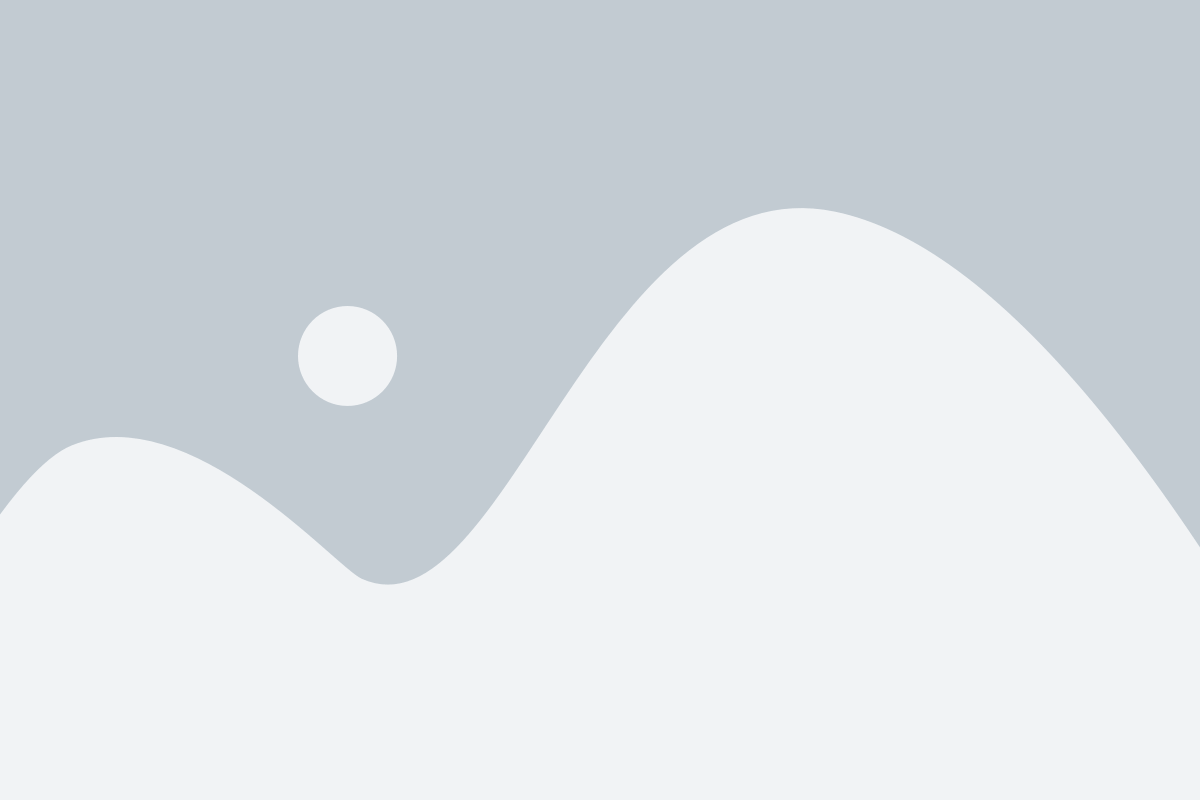
AI = The Future of “H” in HR : Introducing AIQRATE’s consulting offerings for CHRO/CPO/HR leaders
AI = The future of “H” in HR . In today’s competitive businesses , the role of AI in planning, operations & strategy has transformed from being a competitive differentiation to a competitive necessity . The age of “ trust me , this will work” is over. In the current business mandate , where HR is held accountable for delivering business results , it has become imperative to harness the power of AI . AI can elevate HR from a tactical support function to a strategic transformative function . HR business function disruption thru Talent Sciences : business capability of using AI and algorithmic modeling to drive HCM decision making will form the backbone of HR function.
Introducing AIQRATE’s consulting offering for Chief Human Resource Officer (CHRO) / Chief people officer (CPO) / Chief Talent officer (CTO) /HR Leaders working across Enterprises , GCCs , SMBs , Startups , Public Institutions :
- AI master class session : Contextualized for CHRO , CPO : demystify AI , AI strategy canvas , AI landscape & wide applications , HR vale chain interventions
- AI advisor on-demand : Build AI led decision making strategies and processes across the HR value chain and strategic interventions
- AI talent mapping strategies : Execute AIQRATE “T-REX” framework for building enterprise wise AI skilling & learning regime
- AI led interventions for CHRO/CPO : Reimagine HR domain , HR business function problems and scenarios leveraging AIQRATE consulting expertise
- Analytics to AI maturity assessment : Gauge your enterprise AI adoption maturity with AIQRATE “Elevate” transformation journey framework
AIQRATE’s extensive yet bespoke consulting offerings for CHRO/CPO/HR leaders focuses on building AI led strategies on talent workforce decisions and tracking performance of HR strategic initiatives and also on building data driven discovery algorithms on improving HR process efficiencies and outcomes.
AIQRATE’s attempts to gear up HR leaders to the future of work and our curated offerings will enable navigate four broad shifts for HR leaders :
- Accentuate strategic business acumen
2. Augment AI driven expertise for decision making
3. Amplify “transformation driven impact “ within the HR business function.
4. Accelerate “innovation driven culture” within the HR team
Reach out to us at consult@aiqrate.ai for detailed view and approach on our extensive AI consulting offerings for CHRO/CPO/HR leaders .
Related Posts
AIQRATIONS
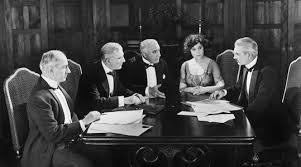
AI led strategy for business transformation : A guided approach for CXOs
Add Your Heading Text Here
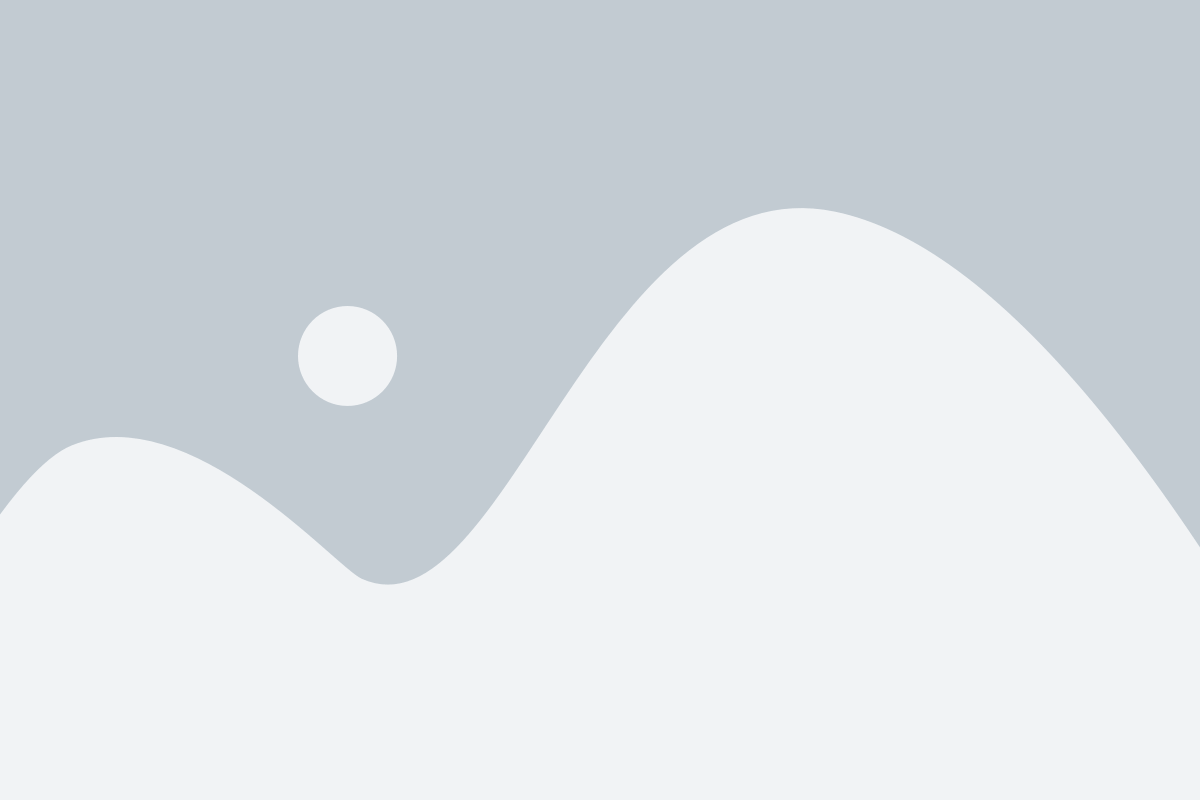
Business transformation programs have long focused on productivity enhancements —taking a “better, faster, cheaper” approach to how the enterprise works. And for good reason: disciplined efforts can boost productivity as well as accountability, transparency, execution, and the pace of decision making. When it comes to delivering fast results to the bottom line, it’s a proven recipe that works.
The problem is, it’s no longer enough. Artificial Intelligence enabled disruption are upending industry after industry, pressuring incumbent companies not only to scratch out stronger financial returns but also to remake who and what they are as enterprises.
Doing the first is hard enough. Tackling the second—changing what your company is and does—requires understanding where the value is shifting in your industry (and in others), spotting opportunities in the inflection points, and taking purposeful actions to seize them. The prospect of doing both jobs at once is sobering.
How realistic is it to think your company can pull it off? The good news is that AIQRATE can demonstrate that it’s entirely possible for organizations to ramp up their bottom-line performance even as they secure game-changing portfolio wins that redefine what a company is and does. What’s more, AL led transformations that focus on the organization’s performance and portfolio appear to load the dice in favor of transformation results. By developing these two complementary sets of muscles, companies can aspire to flex them in a coordinated way, using performance improvements to carry them to the next set of portfolio moves, which in turn creates momentum propelling the company to the next level.
Strategic Steps towards AI led Transformation:
This aspect covers AI led “portfolio-related” moves. The first is active resource reallocation towards building AI led transformation units, which I define as the company shifting more than 20 percent of its capital spending across its businesses or markets over ten years. Such firms create 50 percent more value than counterparts that shift resources at a slower clip.
Meanwhile, a big move in programmatic M&A driven by AI led spot trending—the type of deal making that produces more reliable performance boosts than any other—requires the company to execute at least one deal per year, cumulatively amounting to more than 30 percent of a company’s market capitalization over ten years, and with no single deal being more than 30 percent of its market capitalization.
Making big moves tends to reduce the risk profile and adds more upside than downside. The way I explain this to senior executives is that when you’re parked on the side of a volcano, staying put is your riskiest move.
AI led Transformations that go ‘all in’ by addressing both a company’s performance and its portfolio yield the highest odds.
The implication of these transformation stories is clear: approaches that go all in by addressing both a company’s performance and its portfolio yield the highest odds of lasting improvement. Over the course of a decade, companies that followed this path nearly tripled their likelihood of reaching the top quin tile of the AI transformation power curve relative to the average company in the middle.
Play to win with AI
Life would be simpler if story ended here. However, you’re not operating in a competitive vacuum. As I described earlier, other forces influence your odds of success in significant ways—in particular, how your industry is performing. Research studies have indicated that companies facing competitive headwinds would face longer odds of success than those with tailwinds.
Companies that combined big performance moves with big portfolio moves (including capital expenditures, when not the only portfolio move employed) saw a big lift in their odds. Life is still challenging for these companies—their net odds are dead even—yet this is superior to the negative odds of the other situations.
Winning thru competitive advantage with AI
In an improving industry, the returns to performance improvement are amplified massively. This runs contrary to the very human tendency of equating performance transformations with turnaround cases
The takeaway from all this is that two big rules stand out as commonly and powerfully true whatever your context: first, get moving with AI , don’t be static; second, go all in if you can with AI led transformation programs —it’s always the best outcome (and also the rarest).
Running the AI led transformation program
In my experience, the companies that are most successful at transforming themselves with AI ,sequence their moves so that the rapid lift of performance improvement provides oxygen and confidence for big moves in M&A, capital investment, and resource reallocation. And when the right portfolio moves aren’t immediately available or aren’t clear, the improved performance helps buy a company time until the strategy can catch up.
To illustrate this point, consider the anecdote about Apple that Professor Richard Rumelt describes in his book, Good Strategy/Bad Strategy. It was the late 1990s; Steve Jobs had returned to Apple and cleaned house through productivity-improving cutbacks and a radically simplified product line. Apple was much stronger, yet it remained a niche player in its industry. When Rumelt asked Jobs how he planned to address this fact, Jobs just smiled and said, ‘I am going to wait for the next big thing.’
While no one can guarantee that your “next big thing” will be an iPod-size breakthrough, there’s nothing stopping you from laying the groundwork for a successful AI led transformation. To see how prepared, you are for such an undertaking, ask yourself—and your team—the following five questions. I sincerely hope they provoke productive and transformative discussion among your team.
1.Where is the new business value chain that’s driven by AI
Achieving success with big, portfolio-related moves requires understanding where the business value flows in your business and why. The structural attractiveness of markets, and your position in them, can and does change over time. Ignore this and you might be shifting deck chairs on the Titanic. Meanwhile, to put this thinking into action, you must also view the company as an ever-changing portfolio. This represents a sea change for managers who are used to plodding, once-a-year strategy sessions that are more focused on “getting to yes” and on protecting turf than on debating real alternatives. Get high-powered decision-making algorithms to navigate you thru this transformation.
2. Put your money in building an AI led strategy
Only 10% of the US fortune 200 companies have AI led strategy; this is an impending strategic aspect that cannot be ignored. The dimensions of reimagining customer experience, building innovative products and services and transforming the businesses need to have an AI led strategy move by the CXOs
3.Are you ready for disruption?
Increasingly, incumbent organizations are getting to the pointy end of disruption, where they must accelerate the transition from legacy business models to new ones and even allow potentially cannibalizing businesses to flourish. Sometimes this requires a very deliberate two-speed approach where legacy assets are managed for cash while new businesses are nurtured for growth.
4.Will our company take this seriously?
Embracing AI led transformative change requires commitment, and gaining commitment requires a compelling change story that everyone in the company can embrace. Philips recognized this in 2011 when it launched its “Accelerate” program. Along with productivity improvements and portfolio changes (including a big pivot from electronics to health tech), the company shaped its change story around improving three billion lives annually by 2030, as part of a broader goal of making the world healthier and more sustainable through innovation. Massive thrust and investment was laid by Phillips leadership team on AI led transformation programs.
5.Is the leadership ready for the transformation?
Leading a successful AI led transformation requires a lot more than just picking the right moves and seeing them through. Among your other priorities: build momentum, engage your workforce, and make the change personal for yourself and your company. All of this means developing new leadership skills and ways of working, while embracing a level of commitment as a leader that may be unprecedented for you.
In the end, AI led strategy for transformation is a process and start of a journey …. embrace it or feel the heat of leaving behind. The new age competition is agile and nimble and AI led transformation strategy is a right move to thwart the competition.
Related Posts
AIQRATIONS
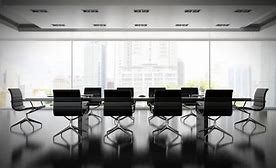
AI led Strategy for Boards : The “new” strategy counselor
Add Your Heading Text Here
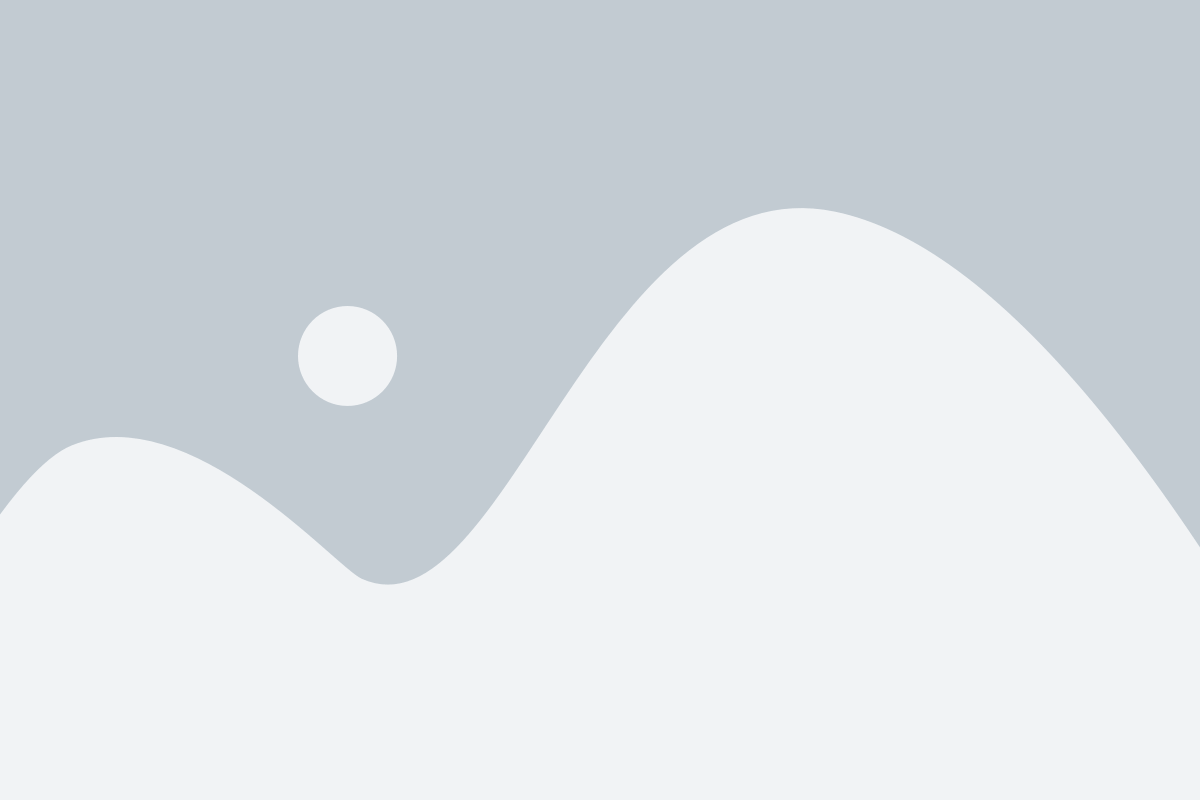
It’s time for boards to craft an AI led strategy . Three strategic aspects can help them and senior leaders to augment decision making process in the board meetings
In the boardroom, and the head of a major global conglomerate is in the hot seat. A director with a background in the manufacturing industry is questioning the economics, an assumption underlying the executive’s industry forecast: that the industry’s ratio of forecast will remain relatively constant. The business leader appears confident about the assumption of stability, which has implications for both the competitive environment and for financial results. But the director isn’t convinced: “In my experience, the forecast changes continuously with the economic cycle and needs to bake in assumptions,” he says, “and I’d feel a whole lot better about these estimates if you had some facts to prove that this has changed.” and the rest of the board doesn’t have it. Finally, the chairman intervenes: “The question being raised is critical and not just for our manufacturing business but for our entire strategy. We’re not going to resolve this today, but let’s make sure it’s covered thoroughly during our strategy off-site and he added , “let’s have some good staff work in place to inform the discussion.”
If the preceding exchange sounds familiar, it should: in the wake of the financial crisis, we find that uncomfortable conversations such as this one are increasingly common in boardrooms around the world as corporate directors and executives come to grips with a changed environment. Ensuring that a company has a great strategy is among a board’s most important functions and the ultimate measure of its stewardship. Yet even as new governance responsibilities and faster competitive shifts require much more—and much better—board engagement on strategy, a great number of boards remain hamstrung by familiar challenges.
Enter AI led strategy for boards
For starters, there’s the problem of time: most boards have about six to eight meetings a year and are often hard pressed to get beyond compliance-related topics to secure the breathing space needed for developing strategy. A recent survey of board members to learn where they’d most like to spend additional time, two out of three picked strategy. A related finding was that 44 percent of directors said their boards simply reviewed and approved management’s proposed strategies. Why such limited engagement? One likely reason is an expertise gap: only 10 percent of the directors felt that they fully understood the industry dynamics in which their companies operated. As a result, only 21 percent of them claimed to have a complete understanding of the current strategy .
What’s more, there’s often a mismatch between the time horizons of board members and of top executives , and that lack of alignment can diminish a board’s ability to engage in well-informed give-and-take about strategic trade-offs. “The chairman of my company has effectively been given a decade,” says the CEO of a company “and I have three years—tops—to make my mark. If I come up with a strategy that looks beyond the current cycle, I can never deliver the results expected from me. Yet I am supposed to work with him to create long-term shareholder value. How am I supposed to make this work?” It’s a fair question, particularly since recent shows that major strategic moves involving active capital reallocation deliver higher shareholder returns than more passive approaches over the long haul, but lower returns over time frames of less than three years.
Compounding these challenges is the increased economic volatility prompting many companies to rethink their strategic rhythm, so that it becomes less calendar driven and formulaic and more a journey involving frequent and regular dialogue among a broader group of executives. To remain relevant, boards must join management on this journey, and management in turn must bring the board along—all while ensuring that strategic co-creation doesn’t become confusion or, worse, shadow management. This is where curating AI strategy for competitive advantage and informed decision making comes to the picture.
Three strategic aspects to ponder on AI led strategy for Boards :
While no one-size-fits-all solution can guide companies as they set out, board members and senior managers ask themselves three simple questions as they approach the development of AI strategy. Using it should raise the quality of decision making , overall engagement and help determine the practical steps each group must take to get there. The usual annual strategic refresh is unlikely to provide the board with an appreciation of the context it would need to address the questions fully, let alone to generate fresh insights in response.
1.Can AI make the boards understand the industry dynamics
Most boards spend most of their strategic time reviewing plans, yet relatively few directors feel they have a complete understanding of the dynamics of the industries their companies operate in or even of how those companies create value. To remedy this problem and to avoid the superficiality it can engender, boards need time—some without management present—so they can more fully understand the structure and economics of the business, as well as how it creates value. They should use this time to get ahead of issues rather than always feeling a step behind during conversations on strategy or accepting management biases or ingrained habits of thought.AI can lay out comprehensive picture of industry and competitive industry dynamics with historical and future forward looking scenarios to make the job of the boards simpler.
2. Can AI trigger enough board–management debate before a specific strategy is discussed?
Aided thru AI and armed with a foundational view based on a clearer understanding of industry and company economics, boards are in a better position to have the kinds of informed dialogue with senior managers that ultimately help them prepare smarter and more refined strategic options for consideration. Board members should approach these discussions with data driven mind-set and with the goal of helping management to broaden its thinking by considering new, even unexpected, perspectives.
During such debates, management’s role is to introduce key pieces of content: a detailed review of competitors, key external trends likely to affect the business, and a view of the specific capabilities the company can use to differentiate itself. The goal of the dialogue is to develop a stronger, shared understanding of the skills and resources the company can use to produce strong returns, as opposed to merely moving with the tide. This is where boards can evangelize and seep in AI in the senior executives group for broader knowledge augmentation .
3.Can AI bring in all strategic options and approaches to the table for board and management ?
Very often, the energizing discussions between the board and management about the business, its economics, and the competition represent the end of the debate. Afterward, the CEO and top team go off to develop a plan that is then presented to the board for approval. Instead, what’s needed at this point is for management to take some time—go thru the self-learning enabled algorithm —to formulate a robust set of strategic options, each followed through to its logical end state, including the implications for the allocation of people, capital, and other resources. These strategic options through the revised algorithmic exercise can then be brought back to the board for discussion and decision making.
Developing AI led strategy is a new phenomenon and will take time to mature —yet will become more powerful algorithmic based decision making process and with board’s increased involvement, which introduces new voices and expertise to the debate and puts pressure on management teams and board members alike to find the best answers. Yet this form of AI led strategy development, when done well, is invaluable. It not only leads to clearer strategies but also creates the alignment necessary to make bolder moves with more confidence and to follow through by committing resources to key decisions. AI led decision making for the boards is here….
(AIQRATE advisory & consulting is a bespoke AI advisory and consulting firm and provide strategic advisory services to boards , CXOs, senior leaders to curate , design building blocks of AI strategy , embed AI@scale interventions and create AI powered enterprises . visit : www.aiqrate.ai )
Related Posts
AIQRATIONS
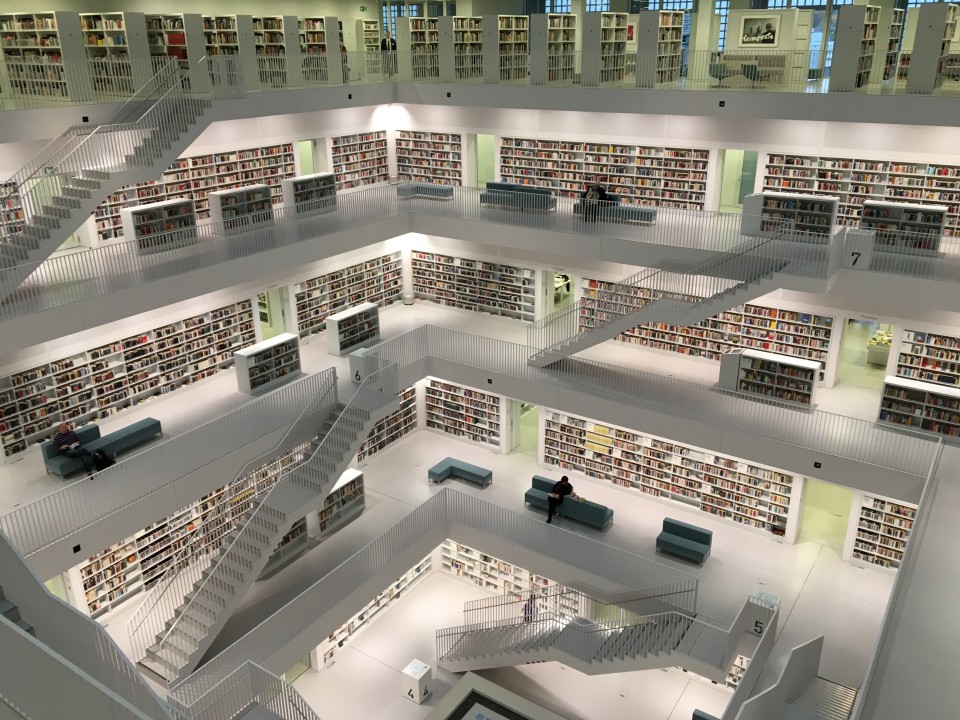
Enterprise Data Engineering Strategy: A must have for New Age organizations
Add Your Heading Text Here
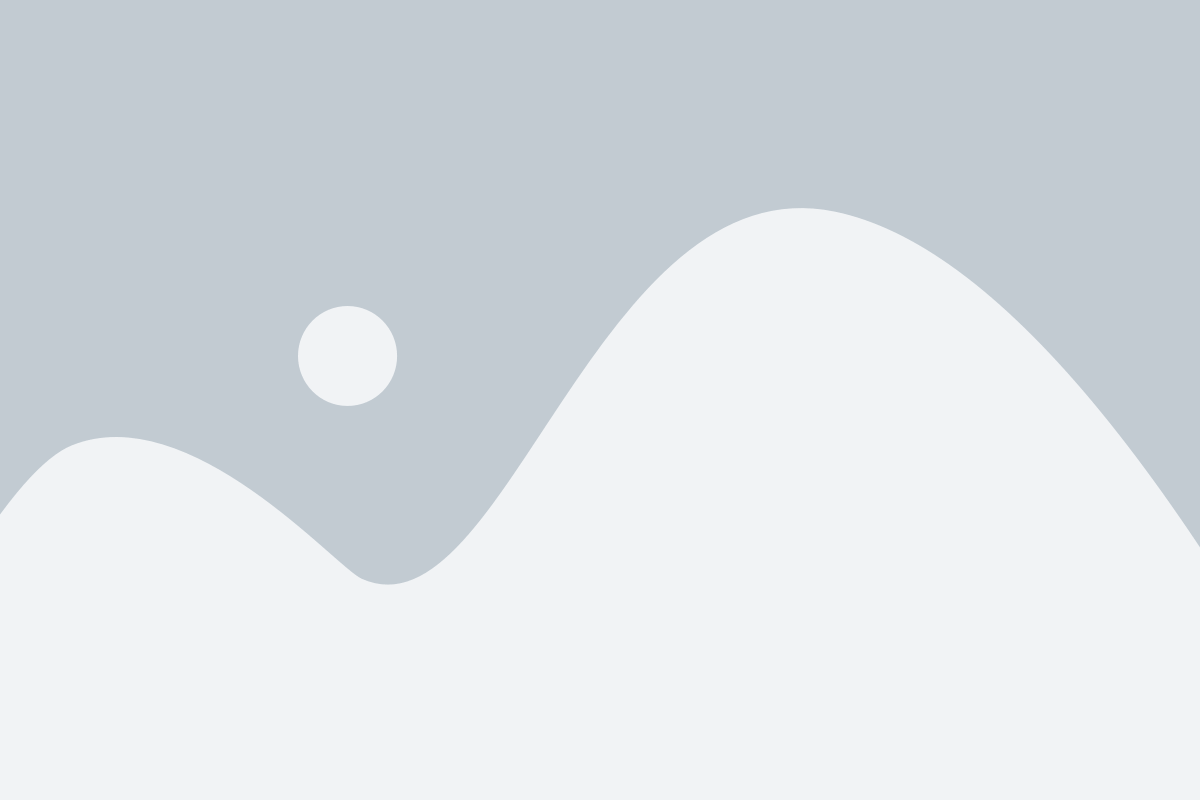
“Enterprise Data Engineering” may sound dated and not so cutting-edge buzzword now. But in this age of several disruptive ideas, concepts and technologies, Enterprise Data Engineering has caught-up with all the necessary advancements and capabilities equally if not better. With organizations becoming more and more keen to be data driven and embrace AI, there is heightened necessity of robust Enterprise Data Engineering Strategy (EDE Strategy). Organizations that do not have efficient EDE Strategy laid out would be ignoring or mismanaging their data asset. Inefficient use of data asset and insights would weaken the competitive edge and their competitors will leap ahead multi-fold in no-time.
EDE Strategy being the master plan of enterprise wide data infrastructure, is a foundational component for any meaningful corporate initiative in recent years. “Explosive growth of data” is being identified as a challenge, an opportunity, a new trend, a significant asset, a source of immense insights, etc. Social media, connected devices and detailed transaction logging are generating huge stream of data which simply can’t be ignored. In simple terms, a panoramic view and wholesome control of a data setup is essential to make data a powerhouse for organizational strategic growth.
Global IP traffic annual run rate is projected to reach 3.3 zettabytes by 2021 (TV & Smartphones together will be accounted for more than 60% of this IP traffic) -CISCO
Along with changing landscape of data, associated disciplines are now aligning to the new demands. EDE Strategy ensures that, these alignments are in line with the roadmap and caters to business users and their requirements.
EDE Strategy encompasses multiple strategy elements that are seeking increased attention now-a-days due to multiple advancements in & around them. Let me introduce some of those strategy elements here:
Automated Data Ingestion & Enrichment
Data Ingestion has now become seriously challenging due to variety & number of sources (social media, new devices, IoT, etc.), new types of data (text stream, video, images, voice, etc), volume of data and the increasing demand for immediately consumable data. Data Ingestion & Enrichment are almost coming together in several scenarios. Due to this, Data Ingestion is almost going off the ETL tools and is fast adopting Pythons and Sparks. Streamed data is not just about absorbing the data as it happens at the source. It is also about enabling AI within the ingestion pipeline to perform data enrichment by bringing together needed data sets (internal & external) and making the Ingestion-to-Insight transformation automatically in seconds/minutes.
ML/AI Models and Algorithms
ML/AI infuses smartness in building data objects, tables, views and models to oversee the data flow across data infrastructure. It uses intelligence in identifying data types/keys/join paths, find & fix data quality issues, identify relationships, identify required data sets to be imported, derive insights, etc. So, the advent of ML/AI in data engineering infuses intelligence into learning, adjusting, alerting and recommending by leaving complex tasks & administration to humans.
Cloud Strategy
The most significant shift seen in the digital world recently is the amount of data being generated and transported. Studies say, 90% of the data existing today were generated in just last 2 years. This is going to increase multi-fold in the coming years. The on-prem based infrastructure and provisioning processes aren’t agile enough to scale rapidly on demand. Even if this is managed, the associated overhead of buying, managing and securing the infrastructure becomes highly expensive and error prone. So, it is essential for organizations to opt for highly efficient and intelligent data platforms on cloud. Cloud offers several advantages across cost, speed, scale, performance, reliability and security. It is also maturing away from initial IaaS into newer services and players. But it doesn’t mean organizations simply initiate the cloud migration and get it done at the press of a button. There should be a carefully drafted Cloud strategy & execution roadmap for adopting cloud in alignment to organization requirements and constraints. Data and information on cloud has the potential to give organizations the flexibility, scalability and ability to discover powerful insights. Cloud also enables applying ML/AI for discovering dark data, monetizing opportunities and disruptive business insights.
Data Lake
Among the data-management technologies most significant space is of data lake. Data Lake is not a specific technology but a concept of housing “one source of truth” data for an organization. When implemented, data lakes can hold and process both structured and unstructured data. Though name indicates huge infrastructure, data lakes are less costly to operate if on cloud. It doesn’t require data to be indexed or prepared to fit specific storage requirements. Instead it holds data in their native formats. Data is then accessed, formatted or reconfigured when needed. Though data lakes are easy to initiate due to easily accessible and affordable cloud offerings, it requires careful planning and incremental adoption model for large scale implementations. In addition, the ever-changing data regulatory & compliance standards add to challenges of implementing and managing data lakes.
Master Data Management (MDM)
Though there is ongoing debate on whether MDM is needed where data lake is the central theme. Schema-on-Write, Schema-on-Read, unstructured data, cloud, etc., are the main contention points in these debates. No matter who wins these debates, it is important for us to know more about MDM while discussing on data engineering. Because, MDM is very essential for organizations to serve their customers/clients near real-time and with better efficiency. As Master Data is the key reference for transactions, typically all independent applications maintain them locally. This leads to redundancy, inconsistency and inefficiency when these data are brought together. It becomes a big challenge while integrating and processing these data due to complexity, chances for errors and increased cost. So, it is important to address MDM element in the EDE strategy carefully by considering organization objectives. Multiple models are practiced for implementing MDM like, registry, hybrid, hub, repository, coexistence, consolidation, etc., which would be discussed in my next articles.
Visualization
Visualization is an exercise that helps in understanding the data in a visual context like patterns, trends, relations, etc. This sounds like an external element or a client to the data engineering. Then, why is Virtualization an important element in EDE Strategy?
Gone are the days of graphs and charts for human analysis. Especially due to data deluge, fitting so much information in a graph or chart is almost impossible for human-beings. They need help in building meaningful representations by consuming huge amount of data. ML/AI is the answer to this wherein its models/algorithms show patterns and correlations by studying huge data-sets in no-time. So, to enable AI to process data, it is important to arrange and label the data suiting it the best. Hence, Visualization is an important aspect to be considered while constructing an Enterprise wide Data Engineering Strategy.
Conclusion
In this new era of data being the new oil, every interest required is being taken to improve the way data is received, cleansed, enriched, assembled and transformed. EDE Strategy ensures establishing effective deployment/management guidelines and continuously improves them because data environments are living organisms. A solid EDE Strategy is highly essential to cater to the demands of this new age. All organizations must have EDE Strategy for realizing their digital vision. Ignoring to have a well laid Enterprise Data Engineering Strategy is as good as regressing in this competitive world.
Related Posts
AIQRATIONS
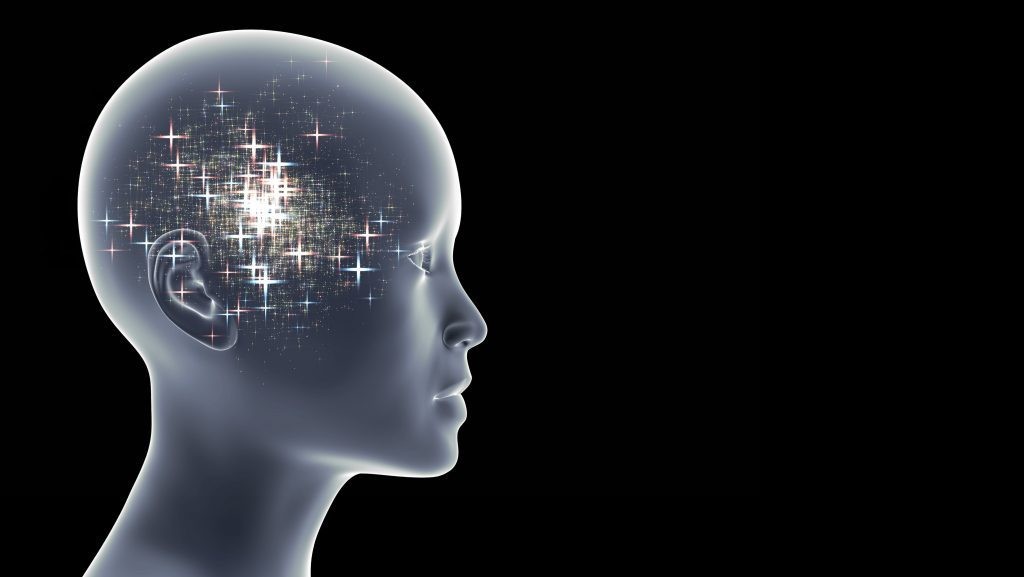
The ‘Dark’ side of AI: Algorithm Bias, influenced decision making.. Defining AI Ethics Strategy
Add Your Heading Text Here
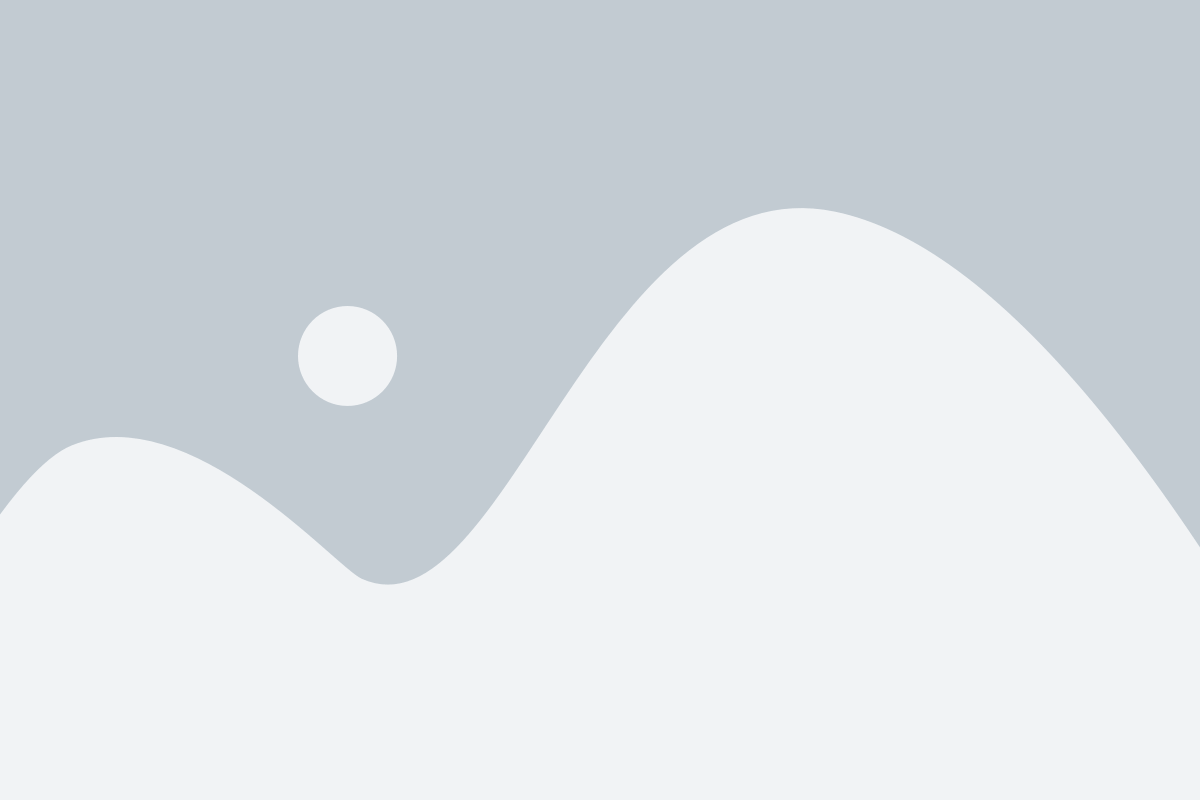
According to a 2019 report from the Centre for the Governance of AI at the University of Oxford, 82% of Americans believe that robots and AI should be carefully managed. Concerns cited ranged from how AI is used in surveillance and in spreading fake content online (known as deep fakes when they include doctored video images and audio generated with help from AI) to cyber attacks, infringements on data privacy, hiring bias, autonomous vehicles, and drones that don’t require a human controller.
What happens when injustices are propagated not by individuals or organizations but by a collection of machines? Lately, there’s been increased attention on the downsides of artificial intelligence and the harms it may produce in our society, from unequitable access to opportunities to the escalation of polarization in our communities. Not surprisingly, there’s been a corresponding rise in discussion around how to regulate AI.
AI has already shown itself very publicly to be capable of bad biases — which can lead to unfair decisions based on attributes that are protected by law. There can be bias in the data inputs, which can be poorly selected, outdated, or skewed in ways that embody our own historical societal prejudices. Most deployed AI systems do not yet embed methods to put data sets to a fairness test or otherwise compensate for problems in the raw material.
There also can be bias in the algorithms themselves and in what features they deem important (or not). For example, companies may vary their product prices based on information about shopping behaviors. If this information ends up being directly correlated to gender or race, then AI is making decisions that could result in a PR nightmare, not to mention legal trouble. As these AI systems scale in use, they amplify any unfairness in them. The decisions these systems output, and which people then comply with, can eventually propagate to the point that biases become global truth.
The unrest on bringing AI Ethics
Of course, individual companies are also weighing in on what kinds of ethical frameworks they will operate under. Microsoft president Brad Smith has written about the need for public regulation and corporate responsibility around facial recognition technology. Google established an AI ethics advisory council board. Earlier this year, Amazon started a collaboration with the National Science While we have yet to reach certain conclusions around tech regulations, the last three years have seen a sharp increase in forums and channels to discuss governance. In the U.S., the Obama administration issued a report in 2016 on preparing for the future of artificial intelligence after holding public workshops examining AI, law, and governance; AI technology, safety, and control; and even the social and economic impacts of AI. The Institute of Electrical and Electronics Engineers (IEEE), an engineering, computing, and technology professional organization that establishes standards for maximizing the reliability of products, put together a crowdsourced global treatise on ethics of autonomous and intelligent systems. In the academic world, the MIT Media Lab and Harvard University established a $27 million initiative on ethics and governance of AI, Stanford is amid a 100-year study of AI, and Carnegie Mellon University established a centre to explore AI ethics.
Corporations are forming their own consortiums to join the conversation. The Partnership on AI to Benefit People and Society was founded by a group of AI researchers representing six of the world’s largest technology companies: Apple, Amazon, DeepMind/Google, Facebook, IBM, and Microsoft. It was established to frame best practices for AI, including constructs for fair, transparent, and accountable AI. It now has more than 80 partner companies. Foundation to fund research to accelerate fairness in AI — although some immediately questioned the potential conflict of interest of having research funded by such a giant player in the field.
Are data regulations around the corner?
There is a need to develop a global perspective on AI ethics, Different societies around the world have very different perspectives on privacy and ethics. Within Europe, for example, U.K. citizens are willing to tolerate video camera monitoring on London’s central High Street, perhaps because of IRA bombings of the past, while Germans are much more privacy oriented, influenced by the former intrusions of East German Stasi spies , in China, the public is tolerant of AI-driven applications like facial recognition and social credit scores at least in part because social order is a key tenet of Confucian moral philosophy. Microsoft’s AI ethics research project involves ethnographic analysis of different cultures, gathered through close observation of behaviours, and advice from external academics such as Erin Meyer of INSEAD. Eventually, we could foresee that there will be a collection of policies about how to use AI and related technologies. Some have already emerged, from avoiding algorithmic bias to model transparency to specific applications like predictive policing.
The longer take is that although AI standards are not top of the line sought after work, they are critical for making AI not only more useful but also safe for consumer use. Given that AI is still young but quickly being embedded into every application that impacts our lives, we could envisage an array of AI ethics guidelines by several countries for AI that are expected to lead to mid- and long-term policy recommendations on AI-related challenges and opportunities.
Chief AI ethical officer on the cards?
As businesses pour resources into designing the next generation of tools and products powered by AI, people are not inclined to assume that these companies will automatically step up to the ethical and legal responsibilities if these systems go awry.
The time when enterprises could simply ask the world to trust artificial intelligence and AI-powered products is long gone. Trust around AI requires fairness, transparency, and accountability. But even AI researchers can’t agree on a single definition of fairness: There’s always a question of who is in the affected groups and what metrics should be used to evaluate, for instance, the impact of bias within the algorithms.
Since organizations have not figured out how to stem the tide of “bad” AI, their next best step is to be a contributor to the conversation. Denying that bad AI exists or fleeing from the discussion isn’t going to make the problem go away. Identifying CXOs who are willing to join in on the dialogue and finding individuals willing to help establish standards are the actions that organizations should be thinking about today. There comes the aspect of Chief AI ethical officer to evangelize, educate, ensure that enterprises are made aware of AI ethics and are bought into it.
When done correctly, AI can offer immeasurable good. It can provide educational interventions to maximize learning in underserved communities, improve health care based on its access to our personal data, and help people do their jobs better and more efficiently. Now is not the time to hinder progress. Instead, it’s the time for enterprises to make a concerted effort to ensure that the design and deployment of AI are fair, transparent, and accountable for all stakeholders — and to be a part of shaping the coming standards and regulations that will make AI work for all
Related Posts
AIQRATIONS
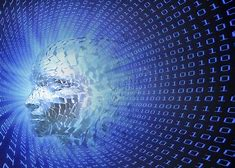
The Eternal Debate: AI – Threat or Opportunity ?
Add Your Heading Text Here
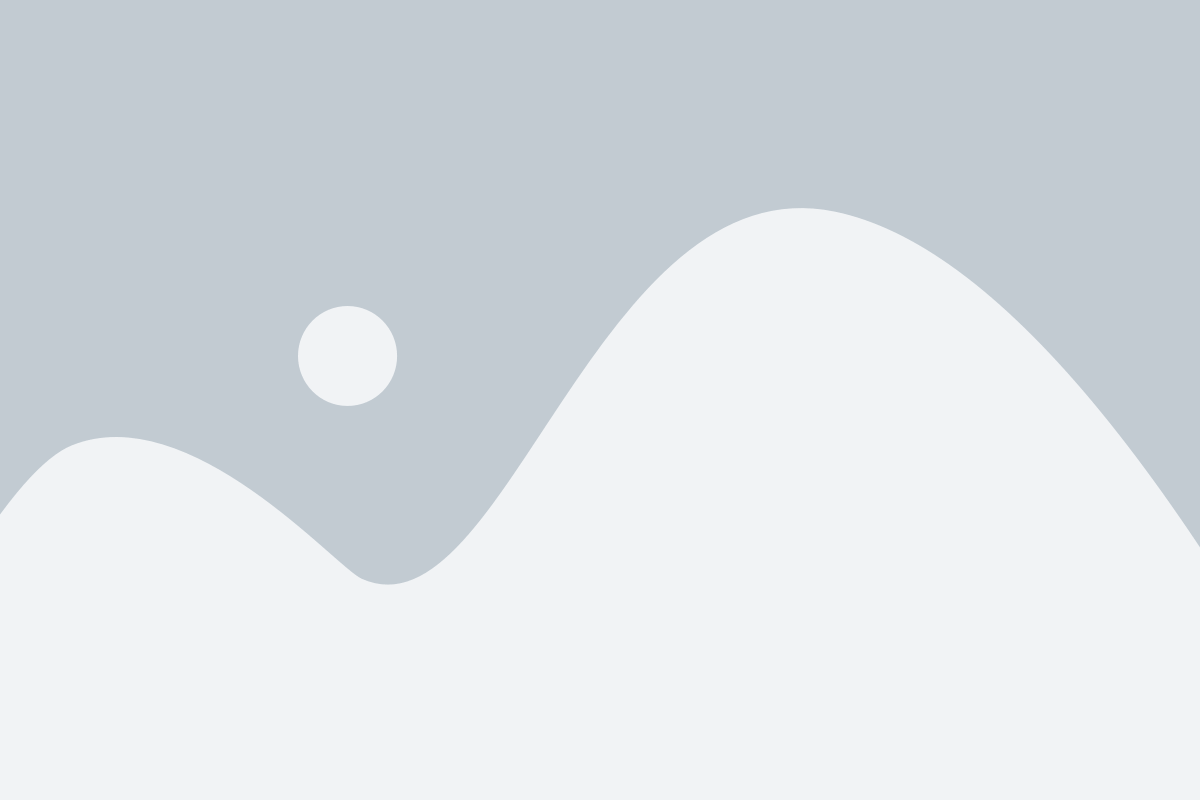
While some predict mass unemployment or all-out war between humans and artificial intelligence, others foresee a less bleak future. A future looks promising, in which humans and intelligent systems are inseparable, bound together in a continual exchange of information and goals, a “symbiotic autonomy.” If you may. It will be hard to distinguish human agency from automated assistance — but neither people nor software will be much use without the other.
Mutual Co-existence – A Symbiotic Autonomy
In the future, I believe that there will be a co-existence between humans and artificial intelligence systems that will be hopefully of service to humanity. These AI systems will involve software systems that handle the digital world, and also systems that move around in physical space, like drones, and robots, and autonomous cars, and also systems that process the physical space, like the Internet of Things.
I don’t think at AI will become an existential threat to humanity. Not that it’s impossible, but we would have to be very stupid to let that happen. Others have claimed that we would have to be very smart to prevent that from happening, but I don’t think it’s true.
If we are smart enough to build machine with super-human intelligence, chances are we will not be stupid enough to give them infinite power to destroy humanity. Also, there is a complete fallacy due to the fact that our only exposure to intelligence is through other humans. There are absolutely no reason that intelligent machines will even want to dominate the world and/or threaten humanity. The will to dominate is a very human one (and only for certain humans).
Even in humans, intelligence is not correlated with a desire for power. In fact, current events tell us that the thirst for power can be excessive (and somewhat successful) in people with limited intelligence.
You will have more intelligent systems in the physical world, too — not just on your cell phone or computer, but physically present around us, processing and sensing information about the physical world and helping us with decisions that include knowing a lot about features of the physical world. As time goes by, we’ll also see these AI systems having an impact on broader problems in society: managing traffic in a big city, for instance; making complex predictions about the climate; supporting humans in the big decisions they have to make.
Intelligence of Accountability
A lot of companies are working hard on making machines to be able to explain themselves — to be accountable for the decisions they make, to be transparent. A lot of the research we do is letting humans or users query the system. When Cobot, my robot, arrives to my office slightly late, a person can ask , “Why are you late?” or “Which route did you take?”
So they are working on the ability for these AI systems to explain themselves, while they learn, while they improve, in order to provide explanations with different levels of detail. People want to interact with these robots in ways that make us humans eventually trust AI systems more. You would like to be able to say, “Why are you saying that?” or “Why are you recommending this?” Providing that explanation is a lot of the research that is being done, and I believe robots being able to do that will lead to better understanding and trust in these AI systems. Eventually, through these interactions, humans are also going to be able to correct the AI systems. So they are trying to incorporate these corrections and have the systems learn from instruction. I think that’s a big part of our ability to coexist with these AI systems.
The Worst Case Contingency
A lot of the bad things humans do to each other are very specific to human nature. Behavior like becoming violent when we feel threatened, being jealous, wanting exclusive access to resources, preferring our next of kin to strangers, etc were built into us by evolution for the survival of the species. Intelligent machines will not have these basic behavior unless we explicitly build these behaviors into them. Why would we?
Also, if someone deliberately builds a dangerous and generally-intelligent AI, other will be able to build a second, narrower AI whose only purpose will be to destroy the first one. If both AIs have access to the same amount of computing resources, the second one will win, just like a tiger a shark or a virus can kill a human of superior intelligence.
In October 2014, Musk ignited a global discussion on the perils of artificial intelligence. Humans might be doomed if we make machines that are smarter than us, Musk warned. He called artificial intelligence our greatest existential threat.
Musk explained that his attempt to sound the alarm on artificial intelligence didn’t have an impact, so he decided to try to develop artificial intelligence in a way that will have a positive affect on humanity
Brain-machine interfaces could overhaul what it means to be human and how we live. Today, technology is implanted in brains in very limited cases, such as to treat Parkinson’s Disease. Musk wants to go farther, creating a robust plug-in for our brains that every human could use. The brain plug-in would connect to the cloud, allowing anyone with a device to immediately share thoughts.
Humans could communicate without having to talk, call, email or text. Colleagues scattered throughout the globe could brainstorm via a mindmeld. Learning would be instantaneous. Entertainment would be any experience we desired. Ideas and experiences could be shared from brain to brain.
We would be living in virtual reality, without having to wear cumbersome goggles. You could re-live a friend’s trip to Antarctica — hearing the sound of penguins, feeling the cold ice — all while your body sits on your couch.
Final Word – Is AI Uncertainty really about AI ?
I think that the research that is being done on autonomous systems — autonomous cars, autonomous robots — it’s a call to humanity to be responsible. In some sense, it has nothing to do with the AI. The technology will be developed. It was invented by us — by humans. It didn’t come from the sky. It’s our own discovery. It’s the human mind that conceived such technology, and it’s up to the human mind also to make good use of it.
I’m optimistic because I really think that humanity is aware that they need to handle this technology carefully. It’s a question of being responsible, just like being responsible with any other technology every conceived, including the potentially devastating ones like nuclear armaments. But the best thing to do is invest in education. Leave the robots alone. The robots will keep getting better, but focus on education, people knowing each other, caring for each other. Caring for the advancement of society. Caring for the advancement of Earth, of nature, improving science. There are so many things we can get involved in as humankind that could make good use of this technology we’re developing
Related Posts
AIQRATIONS
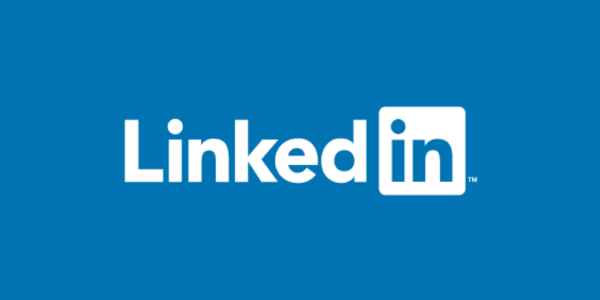
Emerging Roles & Opportunities in Global Capability Centers (GCCs): Enabled by Exponential Technologies
Add Your Heading Text Here
Global Capability Centers(GCC’s) are at a pivotal turning point as the pace at which digitization is changing every aspect is fast paced and agile. The rapid transformation and innovation of GCC’s today is driven by new age or exponential technologies :AI, internet of things (IoT), blockchain, cloud computing , RPA, Cyber security. Exponential technologies are seen to double their performance every couple of years while reducing their costs in half. In recent times; GCC story is in a changing era of value and transformative arbitrage. Most of the GCCs are aiming towards deploying suite of exponential technologies :RPA, Blockchain, IoT, AI to get into digital play. It is widely predicted that exponential technologies will disrupt and transform capability centers in the coming decades.
This blog aims to demystify emerging exponential technologies and examine the developing role that it could play in both the immediate and long-term future of GCC’s. From applying AI to exploring how blockchain could be used to transform businesses, we will envision ways to apply and adopt exponential technologies to GCC related challenges.
Cloud Based Digital Transformation
Big Data technology and cloud computing are widespread across the globe GCC’s are finding the right way to use it, so they can accomplish their business goals. As automation drives businesses, insights derived from big data analytics are like a data mine for businesses to make data-driven decisions. The onset of big data and cloud has led changing job roles and responsibilities in GCC such as BI/BD engineers, Cloud Architects, BI/BD Solutions Architects, Data Visualization Developer.
Automation, RPA for GCC’s
GCC’s today are rapidly adopting robotic process automation. The aim for the workforce is to focus more on value added tasks. Automation value can be leveraged when Cognitive strikes convergence with RPA and enable autonomous decision making, understanding natural language, self-learning and ability to handle scenarios that entail unstructured data and complex decision making.
Automation is seen as the current and huge opportunity in GCC’s. It has a huge potential in its ability to capture the rule-based market. Robotic Process Automation are delivered as virtual Robots, tools, or a set of scripts, an error free enabled automated process. Some of the emerging roles in this area include RPA developer, Deployment engineer
Blockchain
Increased collaboration between businesses, GCC’s and tech vendors unlock the power of blockchain across multiple use cases. Given its immutable and decentralized nature, blockchain will be invaluable in sectors such as manufacturing, supply chain and financial services and we will see innovative use cases coming out of these domains
Within blockchain, smart contracts specifically will gain immense traction. The business value of smart contracts is remarkably clear – they drastically reduce the time and effort for routine but lengthy paperwork processes, while maintaining the sanctity through a blockchain network.
Blockchain development is reshaping the GCC environment with emerging distributed ledger technology. This requires niche skill sets and roles such as Blockchain developers/engineers, Blockchain legal consultant
Artificial Intelligence Predominance
AI’s ability to enhance decision making, reinvent business models and ecosystems, and remake the customer experience will drive the payoff for digital initiatives through 2025. The AI foundation consists of numerous technologies and techniques that have grown over many years: recommendation systems, decision trees, linear regression and neural networks impacting the next-gen GCC’s.
Following core trends in AI will dominate across GCC;s:
Adoption of “plug and play”, as-a-service solutions in AI for organizations with less than global-scale resources to think about integrating narrow AI.
Enterprise Conversational AI will see mainstream adoption and will look to add voice enabled interfaces to their existing point-and-click dashboards and systems.
AI and machine learning continue to be the most penetrable technology trends within GCC’s. The capability centers are adopting software tools that are enabled with machine learning and AI capabilities to eliminate manual intervention. the emerging job titles and roles evolve as Data scientists, Statisticians.
Internet of Things (IoT)
As capability centers are becoming more digital to deliver a connected and seamless experience, IoT will trend among the latest technologies. The emergence of this technologies give rise to newer job roles such as IoT Managers, IoT Business Designers, full stack developers etc. The functional and technical areas of these roles span across the expertise of applying sensors, embedded devices, software and other electronics to businesses with front-end and back-end technologies.
The rise of exponential technologies and the need to stay upbeat with it, allows scope for the changing landscape of GCC’s through new opportunities and roles. Technologies :cloud computing, cyber security , AI , blockchain, robotics process automation (RPA) will continue to be in the fore front of this changing landscape. The GCC’s will continue to directly boost the need for skills on the exponential technologies front . Time for GCC heads and talent acquisition leaders to revamp their business and talent strategies .